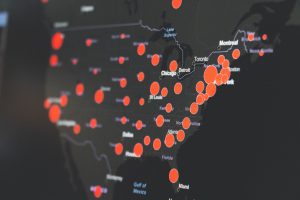
This article was first published by American University in Cairo’s Cairo Review of Global Affairs. It was published as part of UCF’s partnership with AUC, thanks to the generous support of Jonathan and Nancy Wolf.
As of April 4, 2020, the World Health Organization (WHO) has confirmed well over one million global cases of the novel coronavirus—COVID-19. The real number of cases, of course, is likely far higher. As this pandemic continues to claim lives, dominate media coverage, and damage economies, many observers are trying to understand the patterns of this contagion to better understand what to expect and how to evaluate their country’s effectiveness in combating the pathogen. This is particularly true in the United States, where despite difficult access to COVID-19 testing, confirmed cases now number well over two hundred and fifty thousand and appear to be quickly outpacing the number of cases seen in other countries.
A common defense for the skyrocketing numbers in the United States is population size. In other words, given the sheer size of the U.S. population, estimated by the World Bank to sit near 330 million, we should expect far more cases to arise than in a country like Italy, with a population around sixty million. Proponents of this defense will be less concerned with the exponential increase in American cases due to the expectation that it is simply natural.
There is no doubt that this intuition makes sense on its surface. It is both natural and logical to control for population size by dividing the number of known cases of infection by the size of the population, or even creating a count of cases per one hundred thousand people, in order to assess the overall impact of a disease on a country. Such practices are typical for comparative studies and are particularly valuable after an epidemic runs its course. However, it makes very little sense to do this in the early stages of an epidemic for several reasons. Most important is the fact that viral transmissions are not concerned with the size of the overall population, but rather by immediate access to a population that is susceptible to infection.
What does this actually mean for a novel virus like COVID-19? While there are a range of epidemiological models to consider, a common starting point is the expectation of a logistic curve, or an s-curve, as illustrated in the figures below. The line represents the total cumulative cases over time, starting with exponential growth. In other words, the growth rate in the number of cases will be constant over some unit of time, for example, doubling each week. This is what most observers have been paying close attention to: the number of days it takes for COVID-19 infections in a country to double. And because of the limited number of tests, these numbers are ultimately underreported.
Ph.D astrophysicist and senior contributor at Forbes Ethan Siegal’s recent article puts the hazards of exponential growth in stark perspective. Siegal notes, “Exponential growth is so powerful not because it’s necessarily fast, but because it’s relentless,” and “Without introducing a factor to suppress it…is an infectious disease doctor’s nightmare, particularly as more time goes on.” Liz Specht, associate director of science & technology at The Good Food Institute and one of the earlier commentators on COVID-19’s possible trajectory in the United States, used basic statistics to demonstrate how the U.S. healthcare system could become overburdened very quickly. A shortage of hospital beds (the United States has approximately 2.8 per one thousand people) and depleting stockpiles of protective equipment like masks can exacerbate an already difficult and evolving public health crisis.
This is already being seen in multiple respects. Governors have lamented their inability to buy additional supplies. Kentucky Governor Andy Beshear, for example, specifically described a case in which the Federal Emergency Management Agency outbid them for crucial equipment at the last minute. Even testing for the virus has been difficult, with the U.S. Centers for Disease Control and Prevention (CDC) originally distributing an unreliable test and the Trump administration more generally showing very little commitment to increasing access to testing. The lack of domestic capacity was put on full display when South Korea, which in contrast proved to be very capable of mass testing, agreed to Trump’s request to provide support for American test kits.
But beyond concerns of burdening the capacity of health infrastructure in the short term, there is the larger concern of “How bad will it get?”. Particularly concerning is that a new pathogen like COVID-19 has wherever it goes found a population with no prior exposure, which means no immunity. Why is no immunity a problem? No immunity is a problem because every individual offers the virus the potential for a new infection. Each new day will see more people get exposed to the virus, a fraction of those exposures will lead to new infections, and those new cases will then follow suit. Some percentage of those infections will then require hospitalization, and a fraction of those will succumb to the disease. Unsurprisingly, accurate numbers are quite difficult to obtain in real-time, but the CDC’s March 18, 2020 report (covering the period from February12–March 16) reported that at least 12 percent of known cases in the United States required hospitalization.
Explaining Infection Rates
So how does the United States compare to other countries? The answer to this question is best explained through an illustration of how unchecked epidemics are assumed to operate. For the sake of this discussion, we can consider some common starting points in the field of epidemiology and infectious disease. First, assume a first or primary episode of infection starts in two countries at the same time. Second, this case, and those that follow, will typically lead to two other infections. Third, we will assume this will occur within one week. Next, we will assume the rate of infection starts to slow when the vulnerable population is reduced. In other words, the rate slows when more people get infected, limiting the number of potential “new” hosts for the virus.
This is an example of a classic SIR model of epidemiology, which is built on the proportion of a population that is susceptible, infected, or recovered or /removed. As the numbers of infected, recovered (assuming acquired immunity), and removed (through death) increase, the number of susceptible individuals decreases. The people that an infected person exposes themselves to will become less likely to be infected. The pathogen begins to find it difficult to infect new people.
For the purposes of this illustration, we will assume the infection rate drops from two times to 1.5 times when the percentage of the population that has been infected hits 20 percent (or the susceptible population hits 80 percent). During this period, each infection will result in an average of 1.5 new infections. The rate further drops to 1.25 times when the virus has infected 30 percent of the population, one when it hits 40 percent, 0.5 when it hits 50 percent, and 0.25 when it hits 60 percent.
In our example, Country A (population one million) and Country B (population ten million) see their first case of a novel virus in the same week. As the epidemic advances, and as members of the population either recover (with acquired immunity) or pass away, the proportion of the population that is vulnerable dwindles. As a result, the pathogen has a more difficult time finding new people to infect. Rather than each infected individual infecting two new people on average, this rate continues to drop as the vulnerable population decreases. Eventually, the case numbers hit an inflection point, where the rate of increase in infections begins to slow down and, eventually, plateau. At this point, the outbreak effectively ends. This is what herd immunity, a process in which enough people get infected and develop immunity, effectively limiting the number of potential options for the virus, is all about.
The cumulative number of infections in this exercise appears as follows. The curves appear very similar, though Country B has disproportionately more cases due to its larger population. However, closer inspection of the earlier weeks reveals that irrespective of population size, the case numbers actually remain identical.
Whether you are Country A with one million people or Country B with ten million people, the epidemic begins in each country with one case. If we assume the cases originally double in each week, the progression of total cases would start out similarly. One case in the first week, two cases the second week, four cases the third, and so on. Importantly, this progression in absolute numbers does not change based on what the total overall population is—the pathogen still has plenty of potential options to choose from as the entire population is not all exposed at once. It must work its way through it, infection by infection. Regardless of the overall population size, the virus has little difficulty finding susceptible carriers early in the outbreak.
When We Should Start Considering Population Size
However, the total size of the population eventually does matter. We will start to see differences arise when the virus begins to have more difficulty in finding vulnerable targets in Country A (the smaller population). But this takes time, as illustrated in the below figure.
Returning to the concept of exponential growth from each country’s first case in Week 1 and doubling new cases each week, both would see over 131,000 cumulative cases in Week 17. At this point, 13 percent of Country A has been infected, but only 1.3 percent of Country B has been infected. Given these parameters, by Week 18, 20 percent of Country A’s total population will become infected. With the vulnerable population shrinking, new cases ultimately begin to slow.
Country A has now hit its inflection point, where the number of new cases stops accelerating and begins to expand in smaller numbers. Only at this point will we start to expect differences in total cases. With Country B’s original vulnerable population of ten million, the 131,000 cases in Week 17 still leave over 98 percent of Country B’s population vulnerable to infection. While Country A’s new infections quickly begin to decline, Country B’s new cases will continue to double each week until it hits its own inflection point, the 20 percent mark that it will not reach until Week 22.
The result? Country B will ultimately have ten times as many cases as Country A. The epidemic will last three months longer in Country B. Country B’s worst week will arrive when it has nearly one million new infections, almost the entire population of Country A. But for the first seventeen weeks, they would have had the same number of cases.
The United States and Italy
Italy has unfortunately established itself as the grimmest example of COVID-19’s toll. Consequently, it is unsurprising that many would look to Italy for both lessons and comparison. Unfortunately, the United States does not stack up well. The figure reports cumulative case totals for each country by reporting date. Cases appear in green for Italy and blue for the United States. As with the figures above, we see a small number of initial cases slowly transform into a clear exponential increase. While Italy’s case numbers were originally greatly outpacing those of the United States, the latter’s slope continues to grow taller, its numbers approaching those of Italy’s.
However, the lag witnessed here is specifically due to an important difference from the example of the hypotheticals provided above. The outbreak began later in the United States, with the best guess being eleven days later. The red dotted line takes this into account. Whereas before, the United States appeared to have the unfortunate distinction of quickly catching up to Italy, the reality is that the United States has long been ahead of Italy’s pace. And again, this is not due to differences in total population.
Trying to Generalize
If the United State’s numbers look bad this quickly, it is not because it is a country of over three hundred million people. The only exception to this would be comparisons to extremely small polities in which thousands or tens of thousands of cases would have already exhausted the vulnerable population.
The implication of basic models such as this would be that people who are infected in the United States tend to infect other people at a higher rate than what is being seen in other countries. However, this could be due to a variety of issues in the real world. The assumption “all else being equal” that is ubiquitous in basic models, for example, rarely applies. There are differences in hygiene and lifestyle. There are also variations in the number of people that infected individuals expose themselves to. Add to that low government response and the possibilities are endless. Total population size, however, is not a logical explanation for COVID-19 cases in the United States.
It is also likely that progression did not occur only from a single primary case. Instead, dozens—or perhaps even hundreds—of infected individuals likely returned to the United States from abroad, quickly establishing numerous independent clusters across the country. In other words, there is no single “patient zero” in the United States, but many primary infections for independent clusters. But the same is likely true for places like Italy, and even this itself could be seen as the product of slow reaction to the epidemic.
Though in our illustrative example above it is useful to simplify things by assuming a rate of infection doubling each week, this will depend on conditions that ultimately vary in the real world. Perhaps the most easily measurable is population density, as infections in densely populated areas could see more encounters with susceptible people. But while places like New York City are densely populated, the United States as a whole is not. In fact, data from the World Bank shows that its population per square kilometer is less than half that of Italy. In short, national statistics do not work in favor of explaining the U.S. infection rate in terms of national population.
It is also worth pointing out that the parameters in the illustrative example presented here are actually quite conservative. Recall that this approach saw a 20 percent reduction in the vulnerable population begin to quickly slow the spread of new cases, and the outbreak ended after 60 percent of the population was infected. Barring intervention, a team of epidemiological modelers from Imperial College’s COVID-19 response team actually expected this to be far higher, with perhaps over 80 percent of the population facing infection. In short, without interventions such as social distancing, it will be easier for COVID-19 to cause new infections than the hypothetical pathogen reported here.
Further, this exercise reflected two very small populations. The World Bank, for instance, reports that there are at least ninety countries with a population size of at least ten million. Italy and the United States, for example, are well beyond this. The practical implication of this is that it should take longer to see a gap in infections to arise between the two places. Our example again is biased toward an earlier gap between the two countries, and their inflection points would be hit far sooner than in a larger country like the United States.
All else being equal, the tale of the tape may be as follows:
Milestone | Country A (pop: 1 million) |
Country B (pop: 10 million) |
---|---|---|
First case | Week 1 | Week 1 |
10,000 Cases | Week 14 | Week 14 |
100,000 Cases | Week 17 | Week 17 |
500,000 Cases | Week 21 | Week 19 |
1 Million Cases | Never | Week 20 |
5 Million Cases | Never | Week 25 |
Total Cases | 595,455 | 5,766,911 |
Epidemic Ends | Week 31 | Week 46 |
Looking Forward
It is important to note that this exercise largely assumes non- or limited-intervention strategies by governments. And this was indeed the case very early on for several countries, including the United States. Still, while many countries were relatively slow to respond, we have seen sweeping reactive policies ranging from stay at home orders across several U.S. states to nationwide lockdowns in countries like India. South Africa has jailed people who have broken stay at home orders, while Rwanda has reportedly seen such violations result in offenders being killed by the security services.
The effectiveness of such strategies remains to be seen, but the general expectation is that such actions can help slow the rate of transmission. Slowing infections in the short term has the benefit of “flattening the curve,” or reducing the number of cases to a point that it does not overwhelm the healthcare infrastructure. But this also lengthens the amount of time it will take to reduce the susceptible population to a point where the virus has difficulty finding new carriers. This takes time, and it will certainly take far more time than what would be represented by President Trump’s desire to “reopen” the United States in early April. If his suggestions are followed, whatever gains that will have been made in slowing COVID-19’s progression will be quickly unraveled.
As Dr. Antony Fauci stated on ABC News’ program This Week With George Stephanopoulos, “For me, the dynamics and the history of outbreaks is you are never where you think you are…if you think you’re in-line with the outbreak, you’re already three weeks behind. So you’ve got to be almost overreacting a bit to keep up with it.”
Jonathan Powell is a Political Science Professor at UCF.
Chris Faulkner is a professor at Centre College and former fellow of GPII.